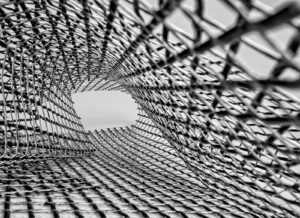
Fraud and financial crime have become extremely complex to detect in the internet era. Criminals can mask their steps through a myriad of layers and parties, very quickly blurring the trail of what funds went where.
Financial crime has always been hard to detect particularly when it moves through unconventional channels, such as terror financing moving through hawala networks. Technologies such as blockchain have only added complexity.
Financial crime was estimated to cost 6% of global GDP, or US$5 trillion in 2019, according to tax and risk advisory firm Crowe. The United Nations Office on Drugs and Crime estimates that US$2 trillion is laundered every year, with $200 million laundered through Australian banks. EY puts the money laundering and associated crime figure as high as US$3.5 trillion.
But financial institutions and law enforcement agencies are starting to fight back with new technologies.
AI can scan vast amounts of data and identify patterns, behaviours, and anomalies faster than humans can. It can also analyse voice records and detect changes in emotion and motivation, which human hearing may not detect, which can give clues about fraudulent activities.
Graph data science is another critical tool. Instead of an old, 2D Excel table, graph databases store data as linked nodes and the relationships between them. This can be used to detect and prevent financial crime by identifying chains and rings of bad actors.
Identifying connections that weren’t obvious
Graph data platform was recently used to help journalists analyse the “data tsunami” of the Pandora Papers. These leaked documents date back to the 1970s and include 11.9 million files totalling 2.94 terabytes of data. The International Consortium of Investigative Journalists (ICIJ) used Neo4j’s graph data platform to generate visualisations and make them searchable.
As ICIJ research editor Emilia Struck explains, graph databases help identify hidden connections that aren’t obvious: “If you find a shareholder or a person, could this person also actually be this person or entity you’ve seen over here, and so be connected to more things I’m not seeing yet. Whenever you have vast amounts of data, your risk is missing what is there. Technology and machine learning, things like graph databases, allow you to see things that sometimes could take you years as a human.”
Platform for banks to fight and prevent fraud
Most major world banks are already using graph data platforms for data lineage, customer 360 and regulatory compliance applications, and some are now starting to use it for fraud detection and anti-money laundering. A key advantage is the ability to detect suspicious activity in real-time. By the time a traditional relational database calculates the complex relationships within a fraud ring, the criminals have already struck and have likely disappeared.
A graph database ensures that relationship-oriented queries are conducted in real-time, so that an anti-fraud team has a chance to strike first.
UBS is using a graph data platform to sift through an overabundance of data and detect potentially suspect connections and carry out more accurate risk analysis. Previously the bank had relied on a system where users had to manually join returned data to glean any data insights. It was complex and costly, with a lot of time wasted sorting through irrelevant data.
Using graph data platforms helped UBS with “data lineage” – this includes data origin, what happens to it and where it moves over time. It gives much more visibility and allows errors – or financial irregularities – to be traced back to the root cause. Putting data into a graph allowed the bank to traverse connected data and make queries much more easily.
At another Fortune 500 financial services company, the fraud detection team was only allowed five minutes to review potentially fraudulent transactions. But just querying the database took eight minutes. With analysts having to review 10,000 daily transactions it wasn’t sustainable. Using a graph database enabled the company to store and traverse several levels of connections and cut the query time to three minutes.
Graph data platform can also help fight cryptocurrency fraud, which amounted to US$1.9 billion in 2020 according to Finaria.it data. Criminals are also increasingly using Bitcoin as a secure, low-cost anonymous way to transfer funds quickly and easily. Cryptocurrencies are typically based on blockchain which timestamps every transaction record in the chain and makes it visible. The entire dataset from the very first to the very last transaction is available to all.
The challenge is in making sense of it all. What does a typical transaction look like compared to an unusual one? With graph data platforms, it’s possible to visualise Bitcoin transactions and identify irregular network activity. Manual transactions (e.g. sending bitcoins to a wallet to pay for goods) look very different to automated transactions (e.g. a cryptocurrency trading bot).
Manual transactions look like small, disconnected components. Automated transactions form huge wheel-shaped patterns. Unusual or irregular patterns may indicate something unusual, or irregular is occurring.
Banks, governments, and corporations generally want to do the right thing and it’s certainly in their interest to follow the law. Financial services firms need to see the value of embracing these new technologies for their due diligence and self-policing to identify bad actors both within their organisation and outside, address faulty processes and practices, minimise risk and losses, and preserve brand trust.
Graph databases are a powerful weapon against the murky world of money laundering and embezzlement, whether from internal employees or sophisticated fraudsters posing as wealthy clients. Fraud rings are continuously growing in shape and size, and traditional tools and methods are of little use in this highly dynamic environment.
But if financial organisations use the right tool for the job, such as graph data platforms, they’ll be able to track and address fraud and fight financial crime in real time.
Nik Vora is the Vice President – APAC at graph data platform provider Neo4j
This article was first published by IT News Asia